- Wamaitha's Newsletter
- Posts
- Optimizing MACD with Genetic Algorithms
Optimizing MACD with Genetic Algorithms
Is the 12,26,9 the best combination for MACD?
Hello Friend!
The experiment of the week is finding the best values for the MACD. Is there a combination that has a better winrate than the 12,26,9? We can use Genetic Algorithms(GA) to find out!
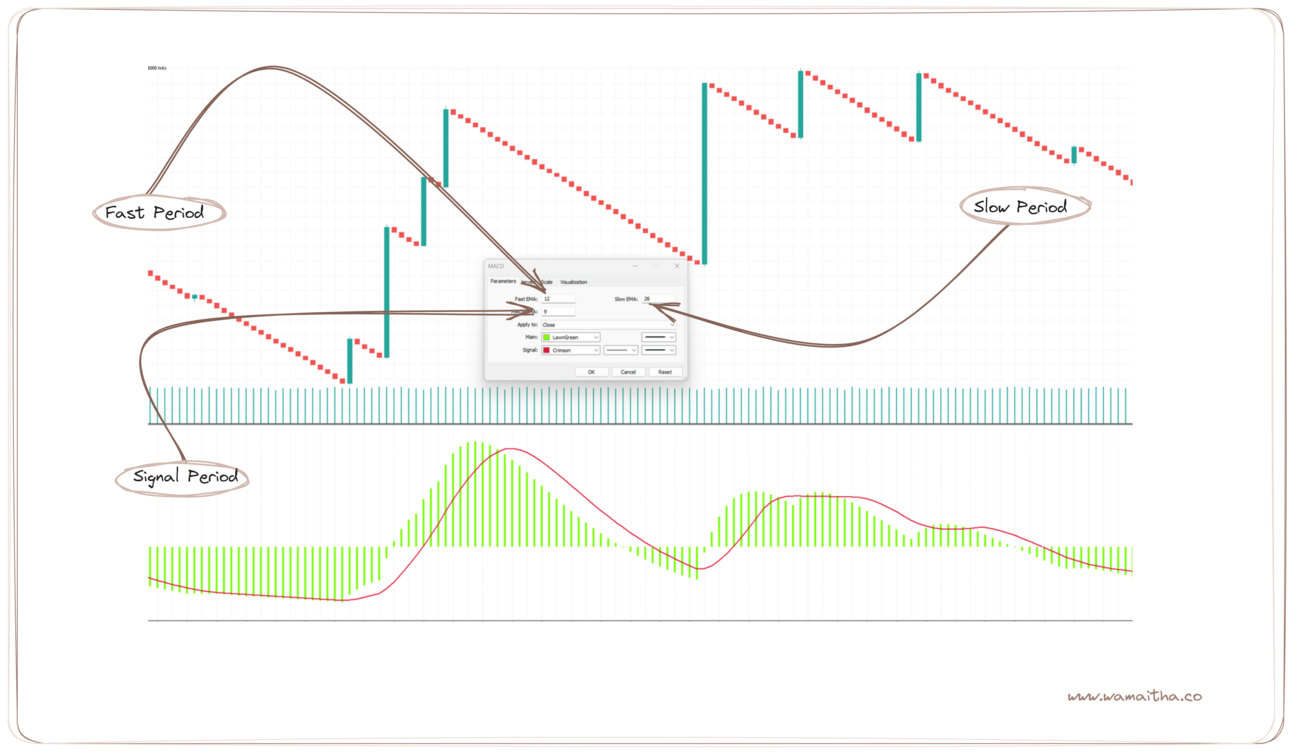
MACD settings can be adjusted but default to 12,26,9
To understand what Genetic Algorithms(GA) are, let’s use an analogy:
Alright, let's say you're playing a video game where you can design your own car to race. You can change the wheels, the body shape, the engine, and the color to make your car faster. However, there are so many options that you're not sure what will make the fastest car. This is where a genetic algorithm (GA) can help!
Start with Random Cars (Initial Population):
Just like in evolution, GAs start with a bunch of random solutions. So, you start with a bunch of randomly designed cars.
Race the Cars (Fitness Evaluation):
Now you race all the cars to see which ones are the fastest. The faster the car, the better it is.
Select the Best Cars (Selection):
You pick the fastest cars because they have the traits (like big engines or aerodynamic shapes) that make them good.
Create Next Generation (Crossover and Mutation):
Now, you mix the traits of these fast cars to create new cars, hoping they'll be even faster. Sometimes, you also change a small thing on a car randomly (like swapping a regular engine for a turbo engine) to see if it makes a car even faster. This is like how in nature, offspring inherit traits from their parents but also have some random changes (mutations).
Repeat:
You keep doing steps 2-4 with the new cars, racing them, selecting the fastest, and making new ones by mixing their traits. Over time, the cars get faster and faster!
In this way, genetic algorithms help find really good solutions to problems by mimicking the process of natural selection. They "evolve" solutions by repeatedly selecting the best ones, mixing them up, and occasionally introducing small random changes, just like how species evolve over time in nature!
Now this applied to the MACD:
Initial Population:
Randomly generate a set of MACD settings (e.g., different values for the short-term EMA, long-term EMA, and signal line EMA periods).
Fitness Evaluation:
Evaluate the performance of these settings on historical market data to determine their "fitness." The fitness could be the total profit, the percentage of winning trades, or some other measure of trading performance.
Selection:
Select the best-performing settings for crossover, favoring those with higher fitness scores.
Crossover:
Combine pairs of selected settings to create a new generation of MACD settings. For example, take the short-term EMA period from one parent and the long-term EMA period from the other parent to create a child setting.
Mutation:
Occasionally, randomly change one or more parameters in the new generation of settings to introduce variety and potentially discover better solutions.
Repeat:
Continue evaluating, selecting, crossing over, and mutating until the MACD settings improve to a satisfactory level or for a set number of generations.
Evaluation:
Once the GA has found optimized MACD settings, these can be evaluated on out-of-sample data to verify their performance.
By using a GA, you're basically allowing the computer to find the most effective MACD settings for a particular trading strategy or market condition, which could potentially enhance your trading performance.
For this experiment, we will closely emulate this paper that optimizes MACD parameters for Soybean Futures. The paper concludes that the new parameters improved the profits generated.
The fitness function will implement the Signal Line Crossover.
Bullish Crossover: When the MACD Line crosses above the Signal Line, it's a signal that the stock may be in an upward trend, suggesting a potential buying opportunity.
Bearish Crossover: Conversely, when the MACD Line crosses below the Signal Line, it indicates a possible downward trend, suggesting a potential selling opportunity.

Who am I?
My name is Wamaitha, a Software Engineer and Content Creator. I am currently building a community on YouTube of traders who want to explore different algorithms applied to finance. Do you fancy that? Give me a follow on YouTube .
Have a trading strategy you need automated? I’m your girl! Fill this form for me and I will get back to you ASAP!
PS: You’re receiving this email because you agreed to receive emails from me.

Thanks for reading! Reply anytime!
Reply